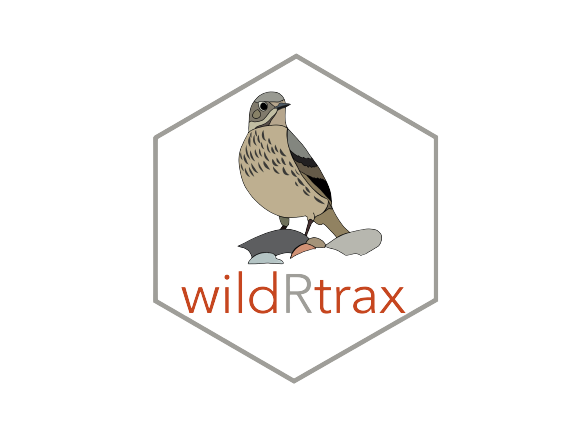
Tutorials
tutorials.Rmd
This vignette provides a series of additional tutorials to help you with using wildRtrax.
Webinars
wildRtrax 1.1.0 Public Webinar
In this webinar, Alex MacPhail, Marcus Becker and Elly Knight, take you on a tour of the main components of the package, including authenticating into WildTrax directly in R, downloading data reports as data frames, and an introduction to various functions that aid in downstream analyses of both ARU and remote camera data.
Acoustic data
Detect missing species using BirdNET
BirdNET is an avian
multi-species classifier. WildTrax utilizes the BirdNET API to
automatically detect species in recordings uploaded to projects once the
task is Transcribed. The results can then be retrieved using
wt_download_report()
:
library(wildRtrax)
Sys.setenv(WT_USERNAME = 'guest', WT_PASSWORD = 'Apple123')
wt_auth()
data <- wt_download_report(620,'ARU',c('birdnet','main'),F)
When downloading multiple reports, the data
object then
becomes a list of tibbles. The results from the main
report
can then be anti-joined against the birdnet
report to
determine if any species were detected than a human did not find. It’s
recommended to use high threshold values from BirdNET and species that
should occur or be allowed in the project.
birdnet_report <- data[[1]] |>
dplyr::filter(is_species_allowed_in_project == 't',
confidence > 50) |>
tibble::add_column(observer = 'BirdNET') |>
dplyr::select(location, recording_date_time, observer, species_code) |>
dplyr::distinct()
main_report <- data[[2]] |>
wt_tidy_species(remove = c("mammal", "amphibian", "abiotic", "insect"), zerofill = F) |>
dplyr::select(location, recording_date_time, observer, species_code) |>
dplyr::distinct()
merged_data <- dplyr::bind_rows(birdnet_report, main_report) |>
dplyr::arrange(location, recording_date_time) |>
dplyr::group_by(location, recording_date_time, observer, species_code) |>
dplyr::mutate(presence = dplyr::row_number()) |>
dplyr::ungroup() |>
tidyr::pivot_wider(names_from = species_code, values_from = presence, values_fill = 0) |>
tidyr::pivot_longer(cols = -c(location, recording_date_time, observer), names_to = "species_code", values_to = "presence")
missing_detections <- merged_data |>
dplyr::filter(observer == "BirdNET" & presence == 1) |>
dplyr::select(location, recording_date_time, species_code) |>
dplyr::anti_join(
merged_data |>
dplyr::filter(observer != "BirdNET") |>
dplyr::select(location, recording_date_time, species_code, presence),
by = c("location", "recording_date_time", "species_code")
)
nrow(missing_detections)
#> [1] 0
Therefore, BirdNET did not detect any additional species.